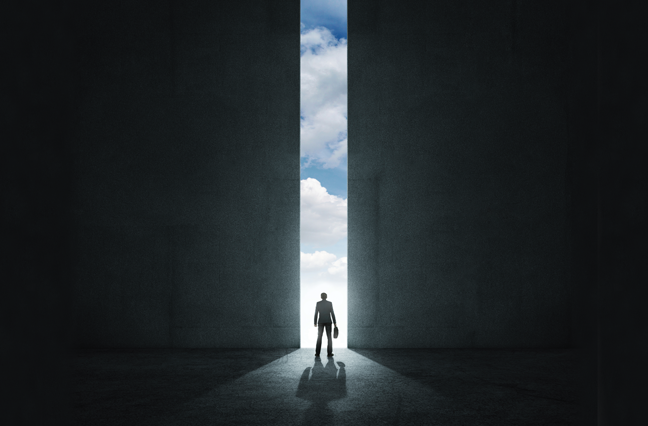
Blind Faith
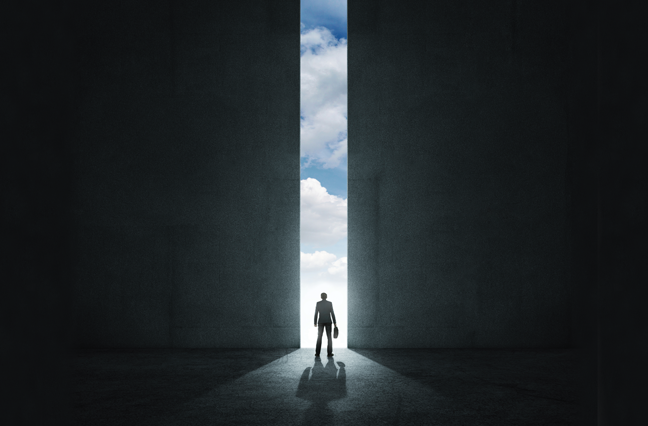
Wouldn’t it be great to be able to predict the future? To know with confidence the way your ball will slice in a gust of wind, about that deer in the road before it’s too late to stop, or just how much capital you need to hold in case of a massive East Coast hurricane.
Such prognosticative skills would make life so much better, success so much easier, and profits so much more predictable. But we all know is that no one can tell the future, right?
Well, maybe we can.
Back in the 1990s, risk carriers began a long-lived love affair with statistically based probabilistic models. They use powerful computing to analyze data and measure exposures for purposes ranging from rate setting to portfolio management and capital allocation.
At the touch of a button, chief underwriting officers can see what risks they hold, how they play off against each other, and how much cash they must have stashed away to survive a worst-case scenario. The modeling process is deeply embedded in the insurance industry, and reliance on it is enormous and increasing.
But can models reveal true exposures and predict possible real losses accurately?
Widespread faith in models is based on a premise known as the “ergodic assumption.” It’s the belief that the future will be just like the past. The modelers’ magic is founded on the hope that a sample of historical data about experiences will match a similar sample of impossible-to-obtain data from the future. Modelers hope to garner, for example, a knowledge of future land-falling hurricanes based on what we know about past hurricanes and how much they cost. Models create a simulacrum—a proxy for future reality. Sometimes it’s right, and sometimes it isn’t. Results can be skewed by the data that go into the model, and since models have a lot of moving parts, accuracy depends heavily on whether those parts are all moving in sync as they should.
Reckless Behavior
There’s another problem, too. As Professor Andrew Cairns of Heriot-Watt University recently explained to a congress of financial risk professionals in Scotland, a properly constructed approximation of future realities can indeed be helpful when making decisions, since it provides another source of insight. However, since model outputs are based on a hypothetical future, plenty of room is left for misinterpretation, misuse and over-reliance. Even if a model is good, it can have bad effects if improperly used. Cairns says the “reckless use of a badly chosen model can lead to disastrous consequences.”
Model problems could strike down an individual insurance company or cut swathes through a sector. Worse, though, is the reality that the widespread use of models has generated a systemic risk, one that cuts across and threatens the entire industry. It has already happened in the investment market, contributing to the international financial crisis. Investors believed they knew the risk of default under mortgage-backed securities because models told them. But later, after the crash, the assumptions surrounding the model were shown to be incorrect. Since the whole sector was using more or less the same models (the ones accepted by credit rating agencies), the process generated a systemic risk. It’s not exaggerating to say that the models caused the global economy to suffer a serious headache.
“Subprime modeling for banks was flawed, but it was also leveraged by the regulators, who said: ‘If you want to sell those mortgage-backed securities, you need a rating from the ratings agencies, and this is the model we expect you to use,’” says J.B. Crozet, head of group underwriting modeling at the London market re/insurer Amlin. “When it was found that the model was wrong and the subprime default rate was way beyond the range of those models, the whole market went at the same time.”
Amlin is concerned about systemic risk to the insurance industry arising from the widespread use of models. To do something about it, the carrier commissioned research from the Future of Humanity Institute at the University of Oxford. The goal of the project is to deepen the understanding of the modeling problem so that attitudes about models change before the whole sector implodes. Amlin has convened an industry working party to grapple with the issue and has published an initial white paper, ominously titled “Systemic Risk of Modelling in Insurance: Did your model tell you all models are wrong?”
The title is a nod to the wisdom of the British statistician George Box, who famously said, “All models are wrong, but some are useful.” But it isn’t just the wrongness of models that’s the problem, Crozet says. Systemic risk, which can be defined as risk that happens in a system because of the way its parts interact rather than faults in the parts themselves, arises from multiple sources inherent in the industry’s use of risk models.
“Some are within the model itself—the risk of the model. Some are behavioral—the underwriters’ attitudes towards models,” Crozet explains. “Some are institutional or organizational—how we treat models and their outputs in the industry.”
Into the latter category falls the prescriptive use of models, as directed by regulators and other agencies.
“Regulators have outsourced regulatory capital-setting and modeling to the companies they regulate, and with that comes a lot of burden on how we have to use models,” Crozet says. Because usage parameters are set and approved by regulators, modeling affects all market players similarly.
“There are more and more of the same models being used within the industry—and within companies as well,” he says, “so we are much more exposed to the systemic risk that one of those models is wrong, that it will affect a lot of insurers at the same time, in quite a dramatic way.”
An example outlined in the white paper is unmodeled losses arising from floods in Thailand in 2011. The inundation caused massive damage to factories manufacturing computer hard drives—Thailand had about a quarter of the global market. Widely used catastrophe models simply didn’t take into account contingent business interruption claims, but in reality knock-on supply-chain problems led to claims under such policies by Japanese manufacturers, massively compounding the overall loss. The final bill was dramatically higher than anyone predicted possible and cost Lloyd’s alone about $2 billion.
Blind Faith
Another, perhaps bigger problem is blind reliance on model outputs. Anders Sandberg, a research fellow at the Future of Humanity Institute, likens this behavior to an aircraft’s autopilot. When it fails, human pilots may not know what to do. “It is easy to become overly dependent,” Sandberg says.
Modeling in insurance can have the same effect, he says. “Ideally everybody will be critically minded and careful and think very deeply about what models are telling them and how they calculated their outputs,” but often they don’t, he says.
This challenge requires model users to think about how they train themselves to use models, how much they look into models, and to ask: Why is it actually saying that? Sometimes the model architecture exacerbates the challenge, since many behave like black boxes. “That’s risky, because you can’t question the output very well,” Sandberg says.
As model users, individuals and companies need to take charge of their own risk. “Many models entice you to take their view of risk,” Sandberg says. “It is the easy way out.” Users need to understand the range within which they can rely on a model and how much they wish to push it, Crozet adds.
This thinking is not new; it is simply relatively uncommon, especially among a generation of underwriters weaned on model outputs. Back in 2007, two years after Hurricane Katrina wreaked havoc on New Orleans, one underwriter explained his view of modeling to investors in Lloyd’s.
“Underlying principles are too often neglected in this computer-driven market,” said Richard Trubshaw, an underwriter of MAP Syndicate 2791. “Some practitioners simply abdicate responsibility to these models and have no idea of how they are made up.” He described as “deeply concerning” the reality that buyers, sellers and intermediaries sometimes base their strategies on a single model with an 80% market share. “This magnifies systemic risk considerably, and the ratings agencies add their pressure to it,” Trubshaw says. If everyone makes the same mistake at the same time, he said, the “resulting market dislocation can be truly catastrophic.”
The danger lurks in the problem that “proprietary modelers keep getting their product wrong,” Trubshaw says. A lack of accounting for claims inflation is one major flaw. Trubshaw’s Lloyd’s syndicate uses its own models, as well as others, to assess risk.
He describes the system as a “theoretical damageability template laid against exposures” and reports the model yielded a maximum foreseeable loss of $93 billion for a Category 5 hurricane hitting Houston, Texas. Lloyd’s internal realistic disaster scenario put the total at $96 billion. “However, one of the largest proprietary modelers has the same event costing only $42 billion and reckons it will happen only once in every 500 years.”
It is not sensible, Trubshaw says, to think that something that happened within the last century will not happen again for 500 years. “The same modelers have consistently produced grotesque underestimates for all the major recent catastrophes,” a shortcoming he described as “delusional exactitude.”
Predicting the Past
The frequency error was highlighted back in 1999, when two large storms, Lothar and Martin, ravaged continental Europe. Storms with such intensity were modeled to be 100- and 30-year events respectively, but they hit within 36 hours of each other. “We now believe Lothar-type events are 30-year events, and Martin-type events seven-year events,” admitted Jacques Blondeau, then chief executive of French reinsurer SCOR, as he announced the hole the systems had blown through the company’s finances that year.
In the case of Lothar and Martin, clearly the catastrophe models fell short when they used data from the past to simulate data from the future. But it wasn’t model outputs alone that caused the insurance industry to take a bath in the wake of the storms. The German weather service gave insufficient warning of Lothar before it arrived. “Partly because Lothar was a very unpredictable weather system,” Sandberg says, “but also because the sensors produced data that were outside the acceptable range.”
The system had a failsafe that removes outliers, and the simulation simply ignored the readings because “it couldn’t be that bad,” Sandberg says. The weather model had far too optimistic a view.
The event reflects a phenomenon that he describes as “asymmetric error correction.” When a model makes a prediction that is expected, users are unlikely to question the output. If something unexpected comes out, they will start looking for problems—and often they will find them. Over time, the model can accumulate the users’ assumptions about the world. In this example, underestimates can go unnoticed while overestimates are expunged, which places a downward bias on the value of the model’s future outputs.
Crozet and Sandberg would be delighted by Trubshaw’s approach. One product of their working party’s discussion of the systemic risk of modeling in insurance is a call for greater use of independent models based on different assumptions or the use of new approaches. They are aware, however, that regulatory challenges can prevent such models from being used or can at least make their application difficult.
Crozet described a colleague’s attempt to reach an independent estimate of a particular terrorist risk. “It was based on a very simple, back-of-the-envelope calculation, and was independent of the elaborate terrorism risk model that his company had been offered.” Despite the very different methodologies behind the two approaches, their loss estimates came down in the same ballpark, Crozet says. “The chance of his estimate and the model both being wrong is much lower than when several models are trained on the same data and work in the same way. Then, there’s a real possibility of all of them being wrong in the same way.”
Sometimes the inaccuracy of models is known but still enforced. For a catastrophe model to be commercially viable in Japan, for example, it must adopt the Japanese government’s official view of earthquake risk, which includes the belief that a Category 9 earthquake isn’t possible in and around Japan. Not everyone agrees, and the 2011 Tohoku earthquake proved the dissenters correct. But until that time, models, which considered the possibility of such an event, did not receive an official seal of approval. “If you had an alternative view of U.S. earthquake risk to that of the U.S. Geological Survey, you would really struggle to sell a model based on it,” Crozet admits.
To get around such problems, Crozet and Sandberg are believers in OASIS, an industry-driven project to create an open marketplace for models and data that could lead to much wider access to understandable tools for catastrophe risk assessment. Supported by major reinsurers, brokers and Lloyd’s, its objectives are to encourage transparency in models, multiply the number available and the sharing of data, and stimulate design innovation, all within a viable commercial framework.
These days the Lloyd’s Market Association is coordinating work to let all Lloyd’s syndicates test the popular Applied Research Associates U.S. windstorm model on the OASIS platform using their own data.
Is There Hope?
Such efforts mark a beginning of attempts to combat the systemic risk of model use. Simon Beale, chief underwriting officer at Amlin, is convinced of the need to change the way insurers use models. The challenge, he says, is to “develop a practical and applied method to consider ways of encouraging the quantification, monitoring, reporting and management of the systemic risk of modeling.”
Professor Ian Goldin, director of the Oxford Martin School at the University of Oxford, says, “Tools that may reduce risk in certain circumstances may have unexpected and destructive ramifications when used unwise…. Understanding how the fallibility of human thinking, competitive pressures, inadequate governance and weak modeling processes interact to produce systemic risk is a first step towards measuring and mitigating it.”
Even after models delivered egregious underestimates of the maximum loss potential for risks ranging from the World Trade Center to the Northridge earthquake to the levee break in New Orleans, voices warning about the systemic risk of modeling are still drowned out, for the most part, by faith-imbued practice.
Of course, it remains impossible to predict the future with certainty, but it is certainly nice to believe we can take a decent shot at it. And the truth is, like the old wisdom about buying IBM, no underwriter will ever be criticized for trying based on what the model says.