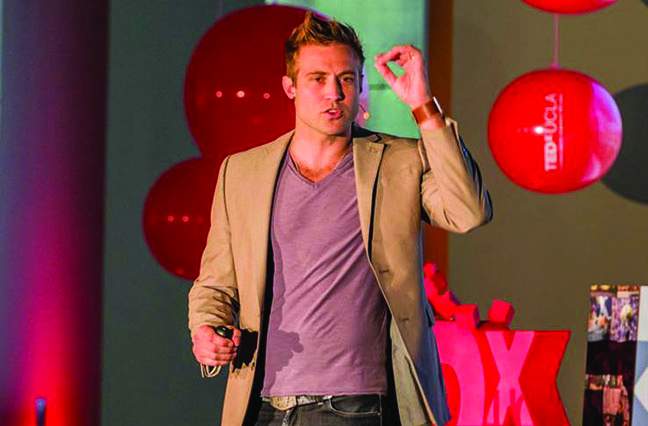
Medical Model
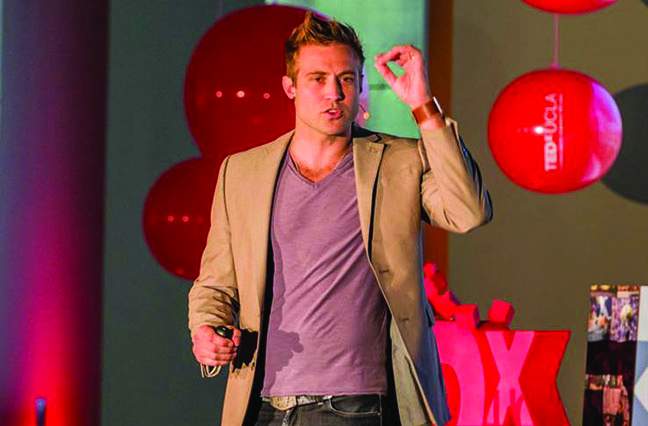
Vaughn conducted research on predictive modeling for patients with inflammatory bowel disease. A multi-disciplinary group was able to predict future hospitalizations and the use of biologics by using insurance data. Their goal was to identify high-risk patients, which would enable providers to offer less-costly treatment up front and avoid high-risk, costly care downstream.
It’s one thing to say you can predict something that is a fun fact. But it’s not useful until you can predict something so well that it actually saves money. Even if it was good for patient care, it has to work within the healthcare economic system we have. You have to prove it can make a difference.
We were surprised that some of these features went together as predictors, because it’s not a human thing to zoom out and look at clusters. We think in terms of narrative and stories, and when you get down to fine and granular aspects, that’s something for machines, not humans.
We use this kind of data on Amazon to predict what people want to buy or Google Maps to predict where someone will go. But it’s not used in medicine. Using data has made such a big impact overall, I have to believe we are leaving something on the table by not using it in this space. We can look at more cases than any doctor can look at in an entire lifetime.
That’s the advantage of using a big-data approach to predictions. This model, used with an interventional treatment plan for high-risk members, could improve outcomes and reduce insurance costs.
We could create interventions where insurers pay for an office visit if it turned out you are at risk: like it was predicted to be highly likely someone would go to the hospital in the next year. Insurers would foot the bill preemptively to prevent that visit from materializing in the first place. Even if an intervention is only 10% effective—meaning it would reduce the risk of hospitalizations only 10% of time—it is still the right call for insurers because hospitals are so incredibly expensive. If you are going to be predicting who is likely to have an adverse medical outcome, you have to predict it so well that it is going to save an insurer money.
And if an insurer foots the bill for someone showing up to the doctor, it lets the patient know the insurance company isn’t trying to get away with whatever they can just to save money. But telling them they are at risk for something and paying for a visit is a wonderful way to have a powerful bond with their insured.
If you zoomed out and looked at the first 30 years of someone’s life, you might be able to predict what they will be doing in the next year. That’s what machines are good at right now.
It’s not necessarily effective now, because data isn’t centralized between hospitals and laboratories and doctors. We thought a good option was to use insurance claims data—that’s services they actually paid for. But you don’t actually get results; it just says, they got an X-ray or are on medications or had this many office visits. We had a nice set of well organized data.
Another difficulty with machine learning is it is sort of looking at things retrospectively. You can’t diagnose what factor is causing something to occur. This type of work we are doing is sort of on the forefront. There’s not even a good model for it.
You can just make a prediction and say someone scores above average on their number of endoscopies, so they may have to look at another type of solution. We can go to the doctor and say, “This person is likely to go to the hospital, and they are not showing any symptoms. Now figure it out.” Maybe they haven’t tried as many medications, so it may be time to shift. Our job is to offer up some predictive model and leave it to the doctors to possibly avert that hospitalization. In the model, doctors start to do preventive medicine.
It’s important to have both doctors and scientists working together on the modeling. When we ran the numbers, we found some things that we thought were really predictive, and the doctors said they wouldn’t be. And we would go back and build the model again. You need multi-disciplinary teams working on this.
But if this does make a difference in the bottom line, they can become an unexpected ally with the potential of overcoming the limitations of the current American healthcare system. They can give us data, and we can look it over, and then the system can do something about it.
With this study, the general science takes much longer because it is the first time anyone has done it. It took a year and a half to get the data and clean it up. It comes in a messy format, and you have to go through and understand what everything means. You are just taking a bunch of data and building a model to see if it is cost effective.
This study establishes a general framework where we built a model from the biggest set of data that we have—from insurers. And you have to run through an economic analysis to make sure it is cost effective for the insurer; otherwise, it won’t happen. There’s always a trade-off for how much we are willing to pay to save people’s lives. Even if you have nationalized healthcare, there is a limit to how much they will spend.