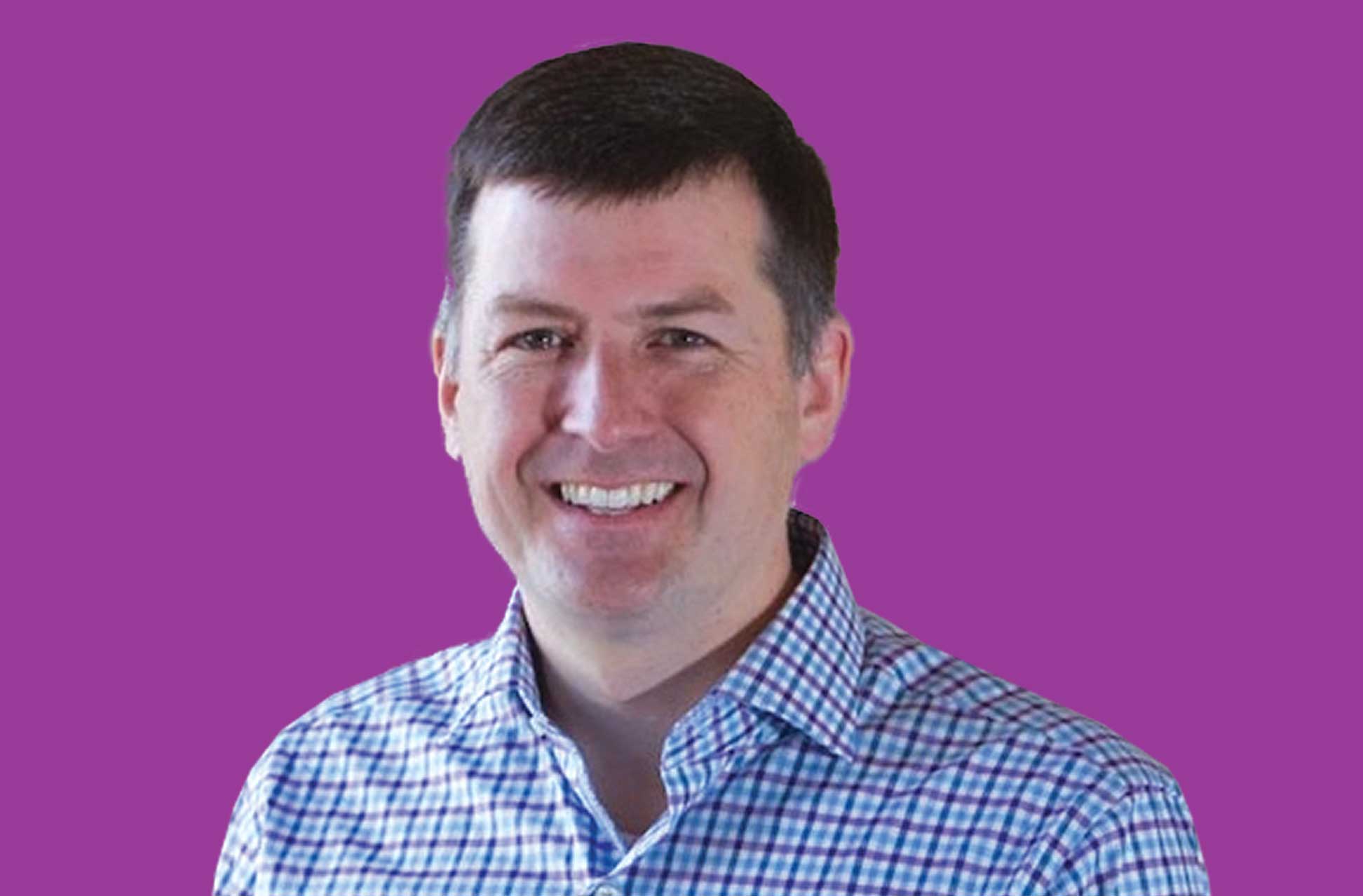
Can Pet Choice Predict Health Risk?
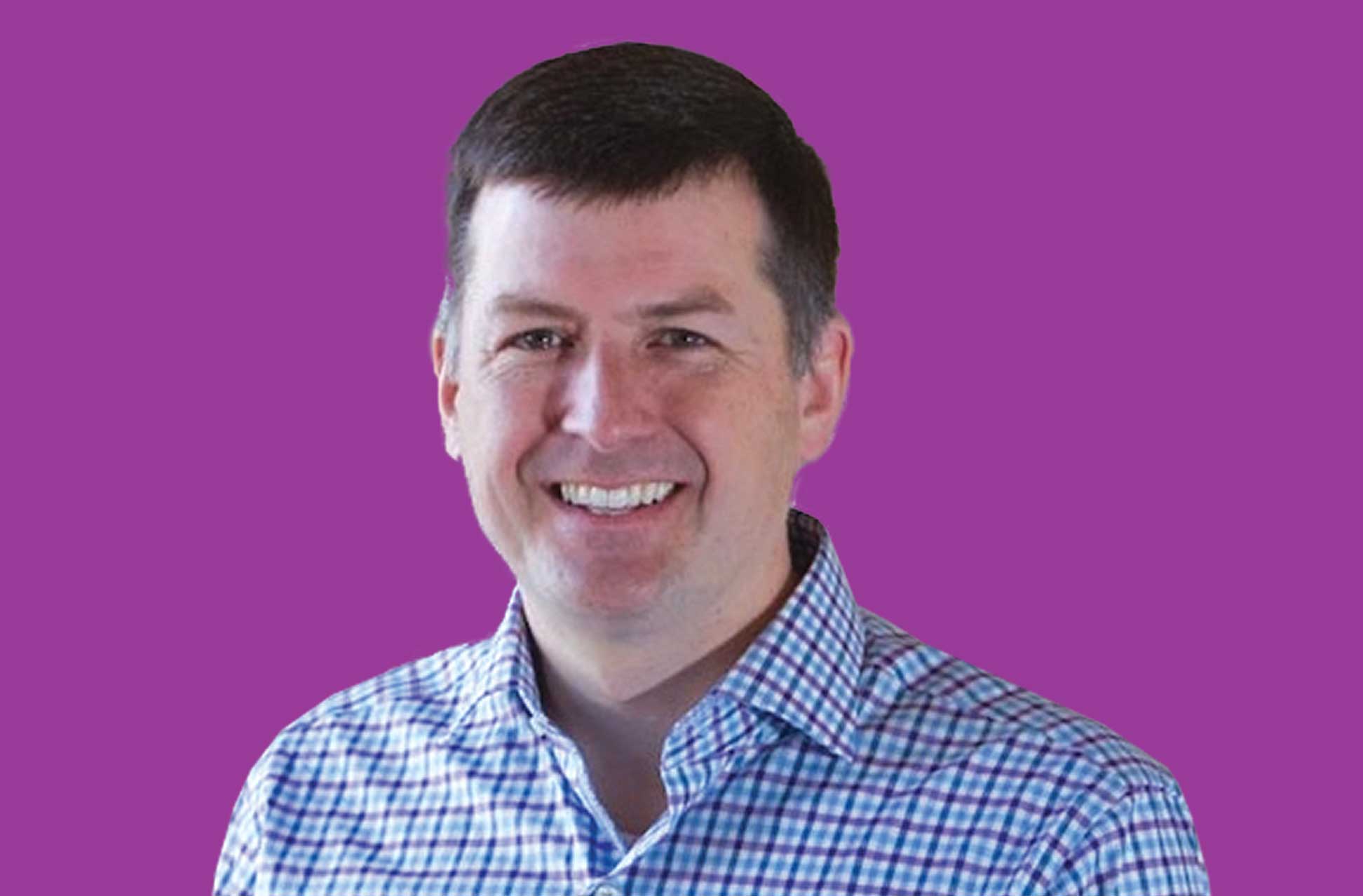
Carrot Health is a Minneapolis-based software firm that creates predictive models with insight into individuals’ health. Waltenbaugh discusses how health data combined with some surprising demographic information can be used to predict health risk and improve outcomes.
We wanted to turn that upside down, starting with the consumer. No one was thinking about the consumer in the way, say, a retailer thinks about who their customers are. I have spent time building companies based on consumer behavioral data and using that to predict what they will do next. It may be determining what product they’ll buy or credential they will achieve, how they learn best, or, in this case, how they will interact with the healthcare world.
We collect data like financial information about individuals, home ownership, educational background, vehicle ownership. We look for markers in purchase patterns and shopping behavior that allow us to forecast things like alcohol consumption and tobacco use and nutrition.
Then we start with a benchmark and can find where a group of employees are over- or under- indexed on risk compared to the population as a whole. It tells us what their risk looks like, and then we score each person on 225 predictive models that forecast their behavior in some way. We can gauge their likelihood to have a chronic illness, follow their doctor’s orders, adhere to a medication regimen, if they have a strong bond with a primary care provider, and a variety of other elements.
Those all put together give us visibility at the individual level on the unique risks and barriers one is facing and suggest their trajectory over the next year or two for improving or worsening their behavior if left alone. Then we suggest where an organization can intervene by offering some next, best actions that should take place.
Statistically, there’s not much to tell them apart, but we can enrich those records, run them through models, and project what their total cost of spend will be over the next few years. In this case, Jim and Matt have a ninefold difference in risk: Jim is likely to spend about $18,000; Matt less than $2,000.
Jim is at high risk of social isolation because he’s divorced, lives alone, he doesn’t spend money on fitness products or travel, he’s a sporadic voter. When we add up those variables and impacts, we find he is less likely to be engaged as a patient and more likely to spiral out of control, not follow doctor’s orders, and worsen his condition.
Matt is married and has support at home. He spends money on fitness and is a regular voter and is a pet owner. Being a pet owner, for diabetics, is associated with better outcomes, and dogs are better than cats. Voters tend to have more connections in the community and more support systems.
Vehicle ownership is another important factor, and it operates in surprising ways. Presence or non-presence of a vehicle, coupled with transit lines and family members nearby, often translates into people who aren’t diligent about seeking care because of access. But different types of vehicles can relate to health, too. For example, minivan ownership can be predictive in a curious way. If a minivan is present in a household with only one or two people, it’s often a sign of obesity. Those cars are the easiest to get in and out of. If there are not children present, they don’t need the cargo capacity of a minivan, so it is correlated with health issues.
Another interesting example is when you look at distances, which become surprisingly predictive. We can tell you someone who lives in an urban area closer to fast food than to a grocery store has a 22% elevated risk of obesity compared to the general population. And people drive only a certain distance to seek primary care. They’ll go much further for specialists or things that are the occasional visit. But for pediatrics or primary care, typically after two to three miles from home, you’ll see a dramatic fall-off in attendance rates.
We have a dashboard for encouraging annual wellness visits, because in each employee population, anywhere from 30% to 40% is not seeking an annual wellness visit. We can identify who is most at risk to skip those early on and then build a list where they have the biggest opportunity to nudge toward that visit, especially for those that we deem at high risk from a clinical perspective.
Clients, at a high level, desire to know more so they can better service their population—to learn what the risk is across the group and what they might be missing and not know about the barriers they are facing. Then they can use that information to craft a better plan.
They also might have a specific intervention they are deploying or have employed that is not working well, and they want to solve it. One group, for instance, identified unplanned ER utilization as a concern. They were trying to figure out how many visits were avoidable, what they could do to change behaviors, and what was causing it.
We know marketing from these firms works, and we know we can counterbalance it by marketing against them. For instance, if you look at smoking in different parts of the country, Delaware is a great example. In that state, if you analyze where discounts are offered on cigarettes, they are only offered in communities that have lower incomes and the least ability to buy cigarettes. Studies have established that in a certain radius of those areas where tobacco is discounted, they correspondingly have the highest smoking rates, which leads to the highest lung cancer rates across the state. Across the country, we are finding areas where people have weaknesses and companies exploit them.
On the other hand, in the state tobacco fund settlements, many states use those funds for anti-smoking campaigns that have brought down smoking rates and given consumers insulation against the marketing from the tobacco companies. This kind of data will give them a fighting chance to change their behaviors and shine a spotlight on what companies are doing to prevent the healthy behaviors from happening. On a much broader scale, it can help people live their best and healthiest lives.